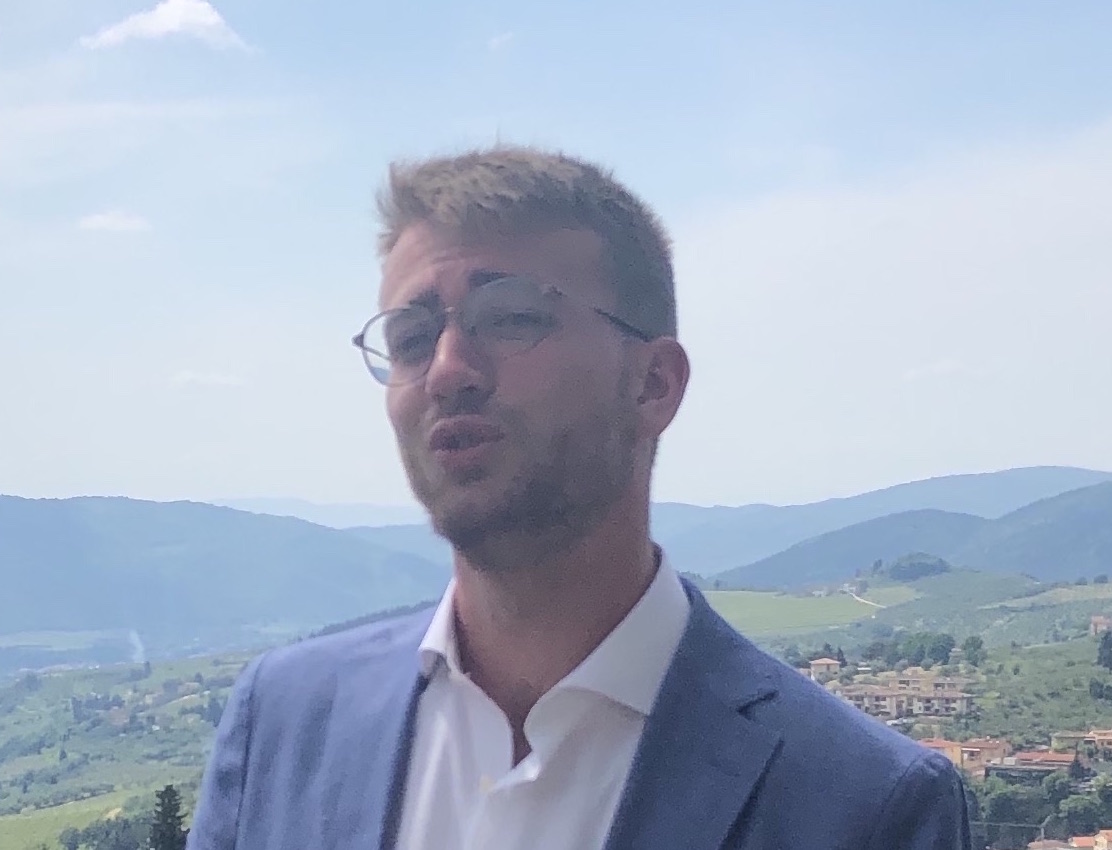
Tuscany, Italy, 2019
About Me
Hello! My name is Owen, I am interested in deep learning. My background is in theoretical condensed matter physics. Outside of science, I enjoy athletics and learning new languages.
- Neural scene representations
- Efficient generative training and inference
Neural Simulation
At the turn of the century, most of the laws of physics that govern the natural world had been established. However, our ability to simulate the full complexity of these physical systems has remained elusive. Neural Network based simulation methods have the potential for fast simulation of physics of complex systems. The ability to simulate large, complex environments requires two key components:
"Holy Grail": The ability to simulate a single human cell at ~2Å resolution
Neural Reasoning
Deep learning methods can now solve IMO/Putnam problems with considerable accuracy. Computer-assisted proofs are an integral part of the mathematician's toolbox. However, there appears to be no reason why neural methods need humans at all.
Can problems in pure mathematics and theoretical physics be solved completely using neural reasoning methods with no human 'hints'? I strongly suspect the answer is yes. Initially, machine learning methods will require significant inductive bias to solve difficult problems. However, as both algorithms and methods scale up, it may be possible to train a single model that can solve pr oblems that have confounded humans since the dawn of history (e.g., the distribution of prime numbers) completely from scratch.
"Holy Grail": A human-verifiable proof of the Riemann Hypothesis, generated via a neural network.